Economic Networks Theory and Computation
Chapter 1 - Introduction (Python Code)
Chapter 1 - Introduction (Python Code)
! pip install --upgrade quantecon_book_networks kaleido
Show code cell output
We begin by importing the quantecon
package as well as some functions and data that have been packaged for release with this text.
import quantecon as qe
import quantecon_book_networks
import quantecon_book_networks.input_output as qbn_io
import quantecon_book_networks.data as qbn_data
import quantecon_book_networks.plotting as qbn_plot
ch1_data = qbn_data.introduction()
default_figsize = (6, 4)
export_figures = False
Next we import some common python libraries.
import numpy as np
import pandas as pd
import networkx as nx
import statsmodels.api as sm
import matplotlib.pyplot as plt
import matplotlib.cm as cm
import matplotlib.ticker as ticker
from mpl_toolkits.mplot3d.art3d import Poly3DCollection
import matplotlib.patches as mpatches
import plotly.graph_objects as go
quantecon_book_networks.config("matplotlib")
Motivation
International trade in crude oil 2021
We begin by loading a NetworkX
directed graph object that represents international trade in crude oil.
DG = ch1_data["crude_oil"]
Next we transform the data to prepare it for display as a Sankey diagram.
nodeid = {}
for ix,nd in enumerate(DG.nodes()):
nodeid[nd] = ix
# Links
source = []
target = []
value = []
for src,tgt in DG.edges():
source.append(nodeid[src])
target.append(nodeid[tgt])
value.append(DG[src][tgt]['weight'])
Finally we produce our plot.
fig = go.Figure(data=[go.Sankey(
node = dict(
pad = 15,
thickness = 20,
line = dict(color = "black", width = 0.5),
label = list(nodeid.keys()),
color = "blue"
),
link = dict(
source = source,
target = target,
value = value
))])
fig.update_layout(title_text="Crude Oil", font_size=10, width=600, height=800)
if export_figures:
fig.write_image("figures/crude_oil_2021.pdf")
fig.show(renderer='svg')
International trade in commercial aircraft during 2019
For this plot we will use a cleaned dataset from Harvard, CID Dataverse.
DG = ch1_data['aircraft_network']
pos = ch1_data['aircraft_network_pos']
We begin by calculating some features of our graph using the NetworkX
and
the quantecon_book_networks
packages.
centrality = nx.eigenvector_centrality(DG)
node_total_exports = qbn_io.node_total_exports(DG)
edge_weights = qbn_io.edge_weights(DG)
Now we convert our graph features to plot features.
node_pos_dict = pos
node_sizes = qbn_io.normalise_weights(node_total_exports,10000)
edge_widths = qbn_io.normalise_weights(edge_weights,10)
node_colors = qbn_io.colorise_weights(list(centrality.values()),color_palette=cm.viridis)
node_to_color = dict(zip(DG.nodes,node_colors))
edge_colors = []
for src,_ in DG.edges:
edge_colors.append(node_to_color[src])
Finally we produce the plot.
fig, ax = plt.subplots(figsize=(10, 10))
ax.axis('off')
nx.draw_networkx_nodes(DG,
node_pos_dict,
node_color=node_colors,
node_size=node_sizes,
linewidths=2,
alpha=0.6,
ax=ax)
nx.draw_networkx_labels(DG,
node_pos_dict,
ax=ax)
nx.draw_networkx_edges(DG,
node_pos_dict,
edge_color=edge_colors,
width=edge_widths,
arrows=True,
arrowsize=20,
ax=ax,
node_size=node_sizes,
connectionstyle='arc3,rad=0.15')
if export_figures:
plt.savefig("figures/commercial_aircraft_2019_1.pdf")
plt.show()
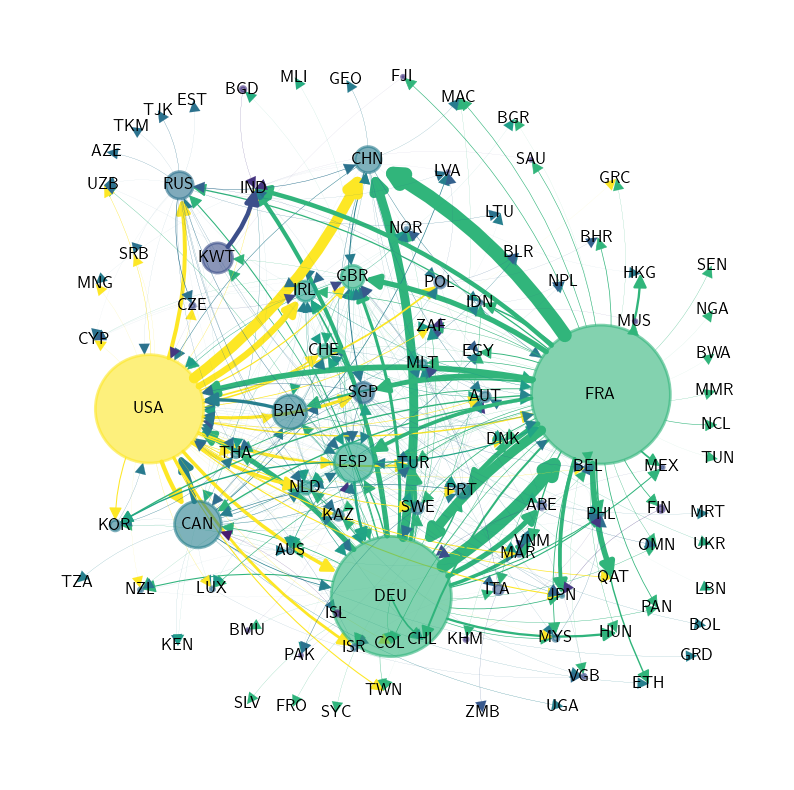
Spectral Theory
Spectral Radii
Here we provide code for computing the spectral radius of a matrix.
def spec_rad(M):
"""
Compute the spectral radius of M.
"""
return np.max(np.abs(np.linalg.eigvals(M)))
M = np.array([[1,2],[2,1]])
spec_rad(M)
3.0000000000000004
This function is available in the quantecon_book_networks
package, along with
several other functions for used repeatedly in the text. Source code for
these functions can be seen here.
qbn_io.spec_rad(M)
3.0000000000000004
Probability
The unit simplex in
Here we define a function for plotting the unit simplex.
def unit_simplex(angle):
fig = plt.figure(figsize=(10, 8))
ax = fig.add_subplot(111, projection='3d')
vtx = [[0, 0, 1],
[0, 1, 0],
[1, 0, 0]]
tri = Poly3DCollection([vtx], color='darkblue', alpha=0.3)
tri.set_facecolor([0.5, 0.5, 1])
ax.add_collection3d(tri)
ax.set(xlim=(0, 1), ylim=(0, 1), zlim=(0, 1),
xticks=(1,), yticks=(1,), zticks=(1,))
ax.set_xticklabels(['$(1, 0, 0)$'], fontsize=16)
ax.set_yticklabels([f'$(0, 1, 0)$'], fontsize=16)
ax.set_zticklabels([f'$(0, 0, 1)$'], fontsize=16)
ax.xaxis.majorTicks[0].set_pad(15)
ax.yaxis.majorTicks[0].set_pad(15)
ax.zaxis.majorTicks[0].set_pad(35)
ax.view_init(30, angle)
# Move axis to origin
ax.xaxis._axinfo['juggled'] = (0, 0, 0)
ax.yaxis._axinfo['juggled'] = (1, 1, 1)
ax.zaxis._axinfo['juggled'] = (2, 2, 0)
ax.grid(False)
return ax
We can now produce the plot.
unit_simplex(50)
if export_figures:
plt.savefig("figures/simplex_1.pdf")
plt.show()
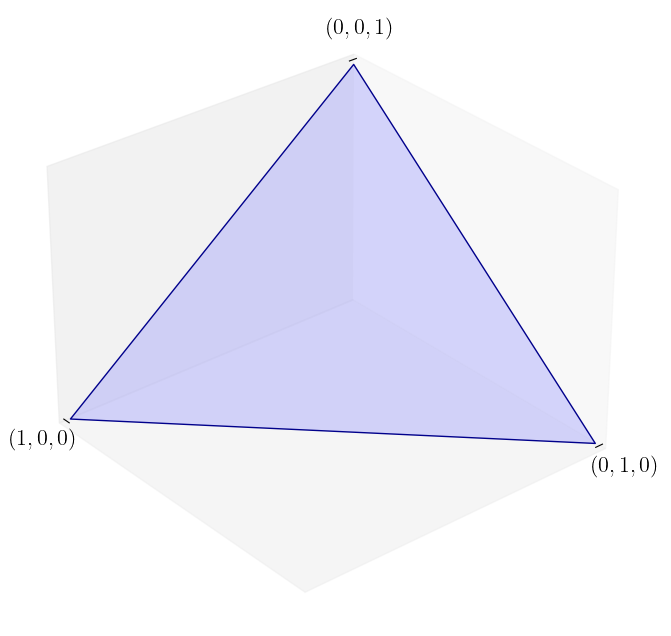
Independent draws from Student’s t and Normal distributions
Here we illustrate the occurrence of “extreme” events in heavy tailed distributions. We start by generating 1,000 samples from a normal distribution and a Student’s t distribution.
from scipy.stats import t
n = 1000
np.random.seed(123)
s = 2
n_data = np.random.randn(n) * s
t_dist = t(df=1.5)
t_data = t_dist.rvs(n)
When we plot our samples, we see the Student’s t distribution frequently generates samples many standard deviations from the mean.
fig, axes = plt.subplots(1, 2, figsize=(8, 3.4))
for ax in axes:
ax.set_ylim((-50, 50))
ax.plot((0, n), (0, 0), 'k-', lw=0.3)
ax = axes[0]
ax.plot(list(range(n)), t_data, linestyle='', marker='o', alpha=0.5, ms=4)
ax.vlines(list(range(n)), 0, t_data, 'k', lw=0.2)
ax.get_xaxis().set_major_formatter(
ticker.FuncFormatter(lambda x, p: format(int(x), ',')))
ax.set_title(f"Student t draws", fontsize=11)
ax = axes[1]
ax.plot(list(range(n)), n_data, linestyle='', marker='o', alpha=0.5, ms=4)
ax.vlines(list(range(n)), 0, n_data, lw=0.2)
ax.get_xaxis().set_major_formatter(
ticker.FuncFormatter(lambda x, p: format(int(x), ',')))
ax.set_title(f"$N(0, \sigma^2)$ with $\sigma = {s}$", fontsize=11)
plt.tight_layout()
if export_figures:
plt.savefig("figures/heavy_tailed_draws.pdf")
plt.show()
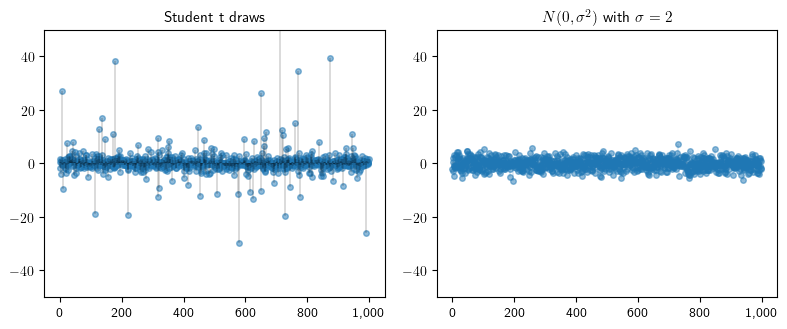
CCDF plots for the Pareto and Exponential distributions
When the Pareto tail property holds, the CCDF is eventually log linear. Here we illustrates this using a Pareto distribution. For comparison, an exponential distribution is also shown. First we define our domain and the Pareto and Exponential distributions.
x = np.linspace(1, 10, 500)
α = 1.5
def Gp(x):
return x**(-α)
λ = 1.0
def Ge(x):
return np.exp(-λ * x)
We then plot our distribution on a log-log scale.
fig, ax = plt.subplots(figsize=default_figsize)
ax.plot(np.log(x), np.log(Gp(x)), label="Pareto")
ax.plot(np.log(x), np.log(Ge(x)), label="Exponential")
ax.legend(fontsize=12, frameon=False, loc="lower left")
ax.set_xlabel("$\ln x$", fontsize=12)
ax.set_ylabel("$\ln G(x)$", fontsize=12)
if export_figures:
plt.savefig("figures/ccdf_comparison_1.pdf")
plt.show()
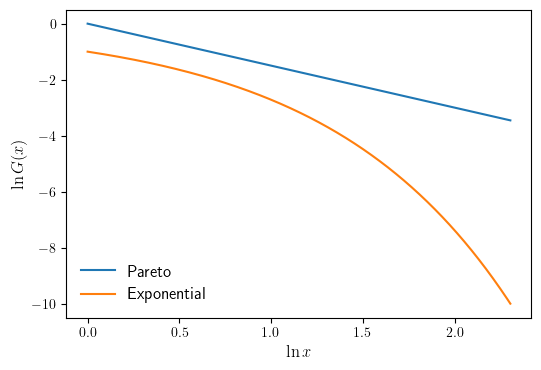
Empirical CCDF plots for largest firms (Forbes)
Here we show that the distribution of firm sizes has a Pareto tail. We start
by loading the forbes_global_2000
dataset.
dfff = ch1_data['forbes_global_2000']
We calculate values of the empirical CCDF.
data = np.asarray(dfff['Market Value'])[0:500]
y_vals = np.empty_like(data, dtype='float64')
n = len(data)
for i, d in enumerate(data):
# record fraction of sample above d
y_vals[i] = np.sum(data >= d) / n
Now we fit a linear trend line (on the log-log scale).
x, y = np.log(data), np.log(y_vals)
results = sm.OLS(y, sm.add_constant(x)).fit()
b, a = results.params
Finally we produce our plot.
fig, ax = plt.subplots(figsize=(7.3, 4))
ax.scatter(x, y, alpha=0.3, label="firm size (market value)")
ax.plot(x, x * a + b, 'k-', alpha=0.6, label=f"slope = ${a: 1.2f}$")
ax.set_xlabel('log value', fontsize=12)
ax.set_ylabel("log prob.", fontsize=12)
ax.legend(loc='lower left', fontsize=12)
if export_figures:
plt.savefig("figures/empirical_powerlaw_plots_firms_forbes.pdf")
plt.show()
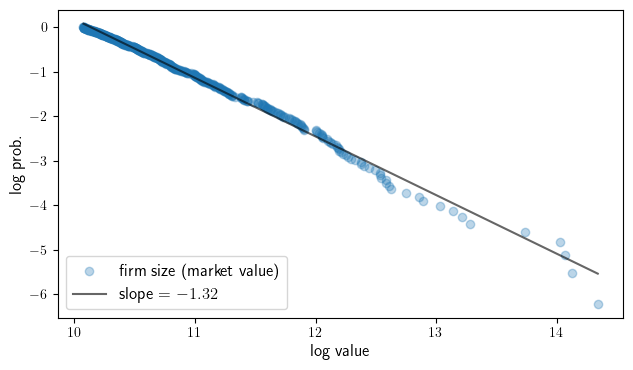
Graph Theory
Zeta and Pareto distributions
We begin by defining the Zeta and Pareto distributions.
γ = 2.0
α = γ - 1
def z(k, c=2.0):
return c * k**(-γ)
k_grid = np.arange(1, 10+1)
def p(x, c=2.0):
return c * x**(-γ)
x_grid = np.linspace(1, 10, 200)
Then we can produce our plot
fig, ax = plt.subplots(figsize=default_figsize)
ax.plot(k_grid, z(k_grid), '-o', label='zeta distribution with $\gamma=2$')
ax.plot(x_grid, p(x_grid), label='density of Pareto with tail index $\\alpha$')
ax.legend(fontsize=12)
ax.set_yticks((0, 1, 2))
if export_figures:
plt.savefig("figures/zeta_1.pdf")
plt.show()
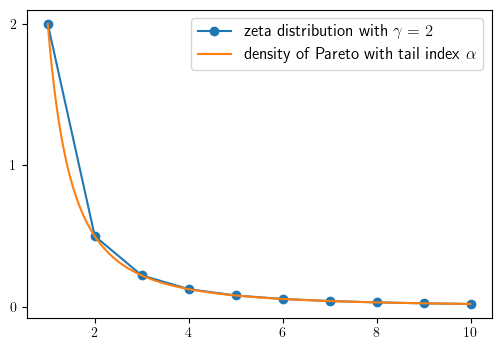
NetworkX digraph plot
We start by creating a graph object and populating it with edges.
G_p = nx.DiGraph()
edge_list = [
('p', 'p'),
('m', 'p'), ('m', 'm'), ('m', 'r'),
('r', 'p'), ('r', 'm'), ('r', 'r')
]
for e in edge_list:
u, v = e
G_p.add_edge(u, v)
Now we can plot our graph.
fig, ax = plt.subplots()
nx.spring_layout(G_p, seed=4)
nx.draw_spring(G_p, ax=ax, node_size=500, with_labels=True,
font_weight='bold', arrows=True, alpha=0.8,
connectionstyle='arc3,rad=0.25', arrowsize=20)
if export_figures:
plt.savefig("figures/networkx_basics_1.pdf")
plt.show()
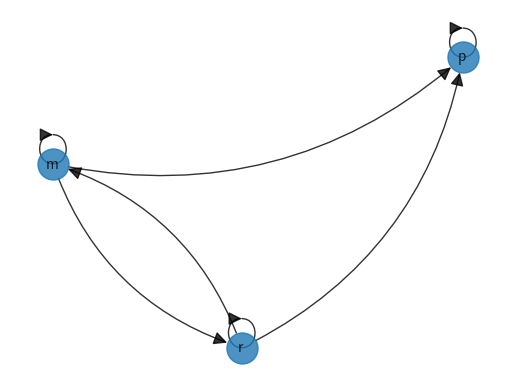
The DiGraph
object has methods that calculate in-degree and out-degree of vertices.
G_p.in_degree('p')
3
G_p.out_degree('p')
1
Additionally, the NetworkX
package supplies functions for testing
communication and strong connectedness, as well as to compute strongly
connected components.
G = nx.DiGraph()
G.add_edge(1, 1)
G.add_edge(2, 1)
G.add_edge(2, 3)
G.add_edge(3, 2)
list(nx.strongly_connected_components(G))
[{1}, {2, 3}]
Like NetworkX
, the Python library quantecon
provides access to some graph-theoretic algorithms.
In the case of QuantEcon’s DiGraph
object, an instance is created via the adjacency matrix.
A = ((1, 0, 0),
(1, 1, 1),
(1, 1, 1))
A = np.array(A) # Convert to NumPy array
G = qe.DiGraph(A)
G.strongly_connected_components
[array([0]), array([1, 2])]
International private credit flows by country
We begin by loading an adjacency matrix of international private credit flows (in the form of a NumPy array and a list of country labels).
Z = ch1_data["adjacency_matrix"]["Z"]
Z_visual= ch1_data["adjacency_matrix"]["Z_visual"]
countries = ch1_data["adjacency_matrix"]["countries"]
To calculate our graph’s properties, we use hub-based eigenvector centrality as our centrality measure for this plot.
centrality = qbn_io.eigenvector_centrality(Z_visual, authority=False)
Now we convert our graph features to plot features.
node_colors = cm.plasma(qbn_io.to_zero_one_beta(centrality))
Finally we produce the plot.
X = qbn_io.to_zero_one_beta(Z.sum(axis=1))
fig, ax = plt.subplots(figsize=(8, 10))
plt.axis("off")
qbn_plot.plot_graph(Z_visual, X, ax, countries,
layout_type='spring',
layout_seed=1234,
node_size_multiple=3000,
edge_size_multiple=0.000006,
tol=0.0,
node_color_list=node_colors)
if export_figures:
plt.savefig("figures/financial_network_analysis_visualization.pdf")
plt.show()
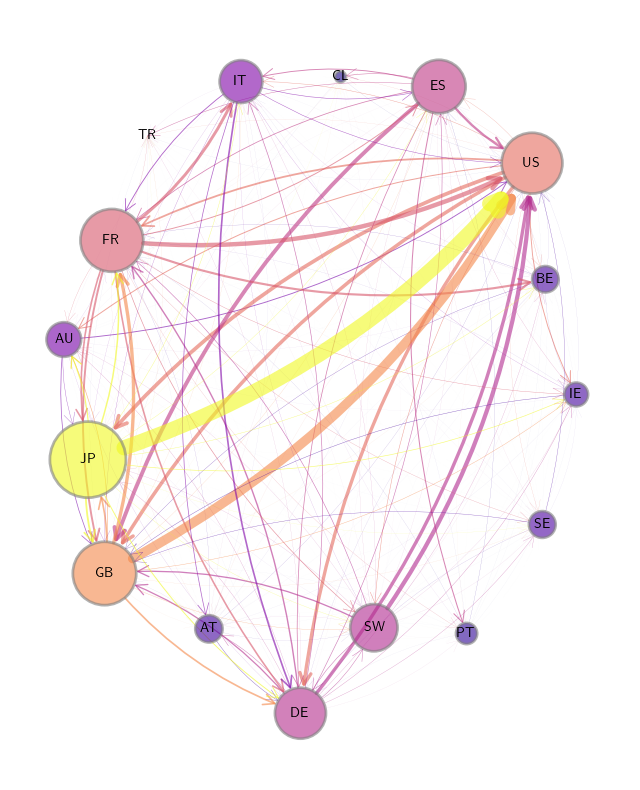
Centrality measures for the credit network
This figure looks at six different centrality measures.
We begin by defining a function for calculating eigenvector centrality.
Hub-based centrality is calculated by default, although authority-based centrality
can be calculated by setting authority=True
.
def eigenvector_centrality(A, k=40, authority=False):
"""
Computes the dominant eigenvector of A. Assumes A is
primitive and uses the power method.
"""
A_temp = A.T if authority else A
n = len(A_temp)
r = spec_rad(A_temp)
e = r**(-k) * (np.linalg.matrix_power(A_temp, k) @ np.ones(n))
return e / np.sum(e)
Here a similar function is defined for calculating Katz centrality.
def katz_centrality(A, b=1, authority=False):
"""
Computes the Katz centrality of A, defined as the x solving
x = 1 + b A x (1 = vector of ones)
Assumes that A is square.
If authority=True, then A is replaced by its transpose.
"""
n = len(A)
I = np.identity(n)
C = I - b * A.T if authority else I - b * A
return np.linalg.solve(C, np.ones(n))
Now we generate an unweighted version of our matrix to help calculate in-degree and out-degree.
D = qbn_io.build_unweighted_matrix(Z)
We now use the above to calculate the six centrality measures.
outdegree = D.sum(axis=1)
ecentral_hub = eigenvector_centrality(Z, authority=False)
kcentral_hub = katz_centrality(Z, b=1/1_700_000)
indegree = D.sum(axis=0)
ecentral_authority = eigenvector_centrality(Z, authority=True)
kcentral_authority = katz_centrality(Z, b=1/1_700_000, authority=True)
Here we provide a helper function that returns a DataFrame for each measure. The DataFrame is ordered by that measure and contains color information.
def centrality_plot_data(countries, centrality_measures):
df = pd.DataFrame({'code': countries,
'centrality':centrality_measures,
'color': qbn_io.colorise_weights(centrality_measures).tolist()
})
return df.sort_values('centrality')
Finally, we plot the various centrality measures.
centrality_measures = [outdegree, indegree,
ecentral_hub, ecentral_authority,
kcentral_hub, kcentral_authority]
ylabels = ['out degree', 'in degree',
'eigenvector hub','eigenvector authority',
'Katz hub', 'Katz authority']
ylims = [(0, 20), (0, 20),
None, None,
None, None]
fig, axes = plt.subplots(3, 2, figsize=(10, 12))
axes = axes.flatten()
for i, ax in enumerate(axes):
df = centrality_plot_data(countries, centrality_measures[i])
ax.bar('code', 'centrality', data=df, color=df["color"], alpha=0.6)
patch = mpatches.Patch(color=None, label=ylabels[i], visible=False)
ax.legend(handles=[patch], fontsize=12, loc="upper left", handlelength=0, frameon=False)
if ylims[i] is not None:
ax.set_ylim(ylims[i])
if export_figures:
plt.savefig("figures/financial_network_analysis_centrality.pdf")
plt.show()
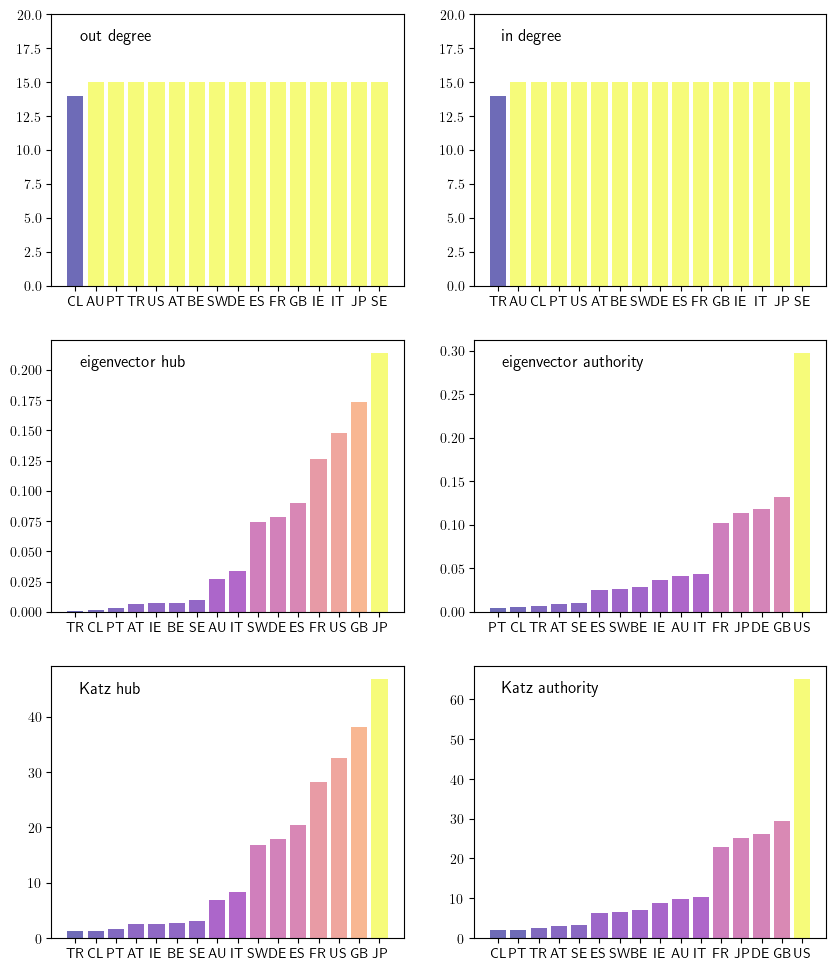
Computing in and out degree distributions
. The in-degree distribution of a NetworkX
DiGraph can be calculated using the code below.
def in_degree_dist(G):
n = G.number_of_nodes()
iG = np.array([G.in_degree(v) for v in G.nodes()])
d = [np.mean(iG == k) for k in range(n+1)]
return d
The out-degree distribution is defined analogously.
def out_degree_dist(G):
n = G.number_of_nodes()
oG = np.array([G.out_degree(v) for v in G.nodes()])
d = [np.mean(oG == k) for k in range(n+1)]
return d
Degree distribution for international aircraft trade
Here we illustrate that the commercial aircraft international trade network is approximately scale-free by plotting the degree distribution alongside with and.
In this calculation of the degree distribution, performed by the NetworkX
function degree_histogram
, directions are ignored and the network is treated
as an undirected graph.
def plot_degree_dist(G, ax, loglog=True, label=None):
"Plot the degree distribution of a graph G on axis ax."
dd = [x for x in nx.degree_histogram(G) if x > 0]
dd = np.array(dd) / np.sum(dd) # normalize
if loglog:
ax.loglog(dd, '-o', lw=0.5, label=label)
else:
ax.plot(dd, '-o', lw=0.5, label=label)
fig, ax = plt.subplots(figsize=default_figsize)
plot_degree_dist(DG, ax, loglog=False, label='degree distribution')
xg = np.linspace(0.5, 25, 250)
ax.plot(xg, 0.2 * xg**(-1.1), label='power law')
ax.set_xlim(0.9, 22)
ax.set_ylim(0, 0.25)
ax.legend()
if export_figures:
plt.savefig("figures/commercial_aircraft_2019_2.pdf")
plt.show()
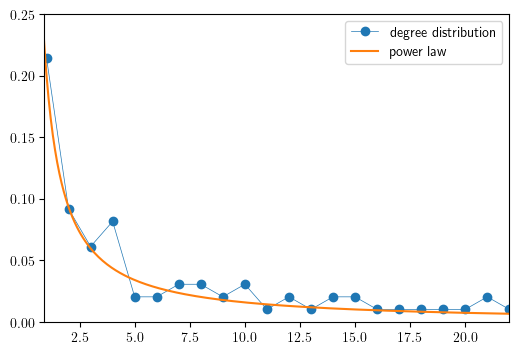
Random graphs
itertools
library. The function
combinations(A, k)
returns a list of all subsets of of size . For example:
import itertools
letters = 'a', 'b', 'c'
list(itertools.combinations(letters, 2))
[('a', 'b'), ('a', 'c'), ('b', 'c')]
Below we generate random graphs using the Erdos-Renyi and Barabasi-Albert algorithms. Here, for convenience, we will define a function to plot these graphs.
def plot_random_graph(RG,ax):
node_pos_dict = nx.spring_layout(RG, k=1.1)
centrality = nx.degree_centrality(RG)
node_color_list = qbn_io.colorise_weights(list(centrality.values()))
edge_color_list = []
for i in range(n):
for j in range(n):
edge_color_list.append(node_color_list[i])
nx.draw_networkx_nodes(RG,
node_pos_dict,
node_color=node_color_list,
edgecolors='grey',
node_size=100,
linewidths=2,
alpha=0.8,
ax=ax)
nx.draw_networkx_edges(RG,
node_pos_dict,
edge_color=edge_colors,
alpha=0.4,
ax=ax)
An instance of an Erdos–Renyi random graph
n = 100
p = 0.05
G_er = qbn_io.erdos_renyi_graph(n, p, seed=1234)
fig, axes = plt.subplots(1, 2, figsize=(10, 3.2))
axes[0].set_title("Graph visualization")
plot_random_graph(G_er,axes[0])
axes[1].set_title("Degree distribution")
plot_degree_dist(G_er, axes[1], loglog=False)
if export_figures:
plt.savefig("figures/rand_graph_experiments_1.pdf")
plt.show()
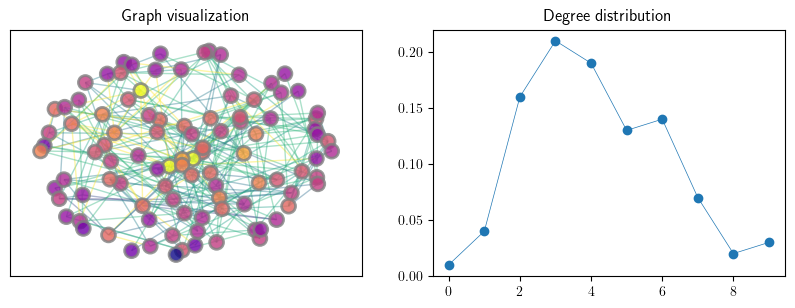
An instance of a preferential attachment random graph
n = 100
m = 5
G_ba = nx.generators.random_graphs.barabasi_albert_graph(n, m, seed=123)
fig, axes = plt.subplots(1, 2, figsize=(10, 3.2))
axes[0].set_title("Graph visualization")
plot_random_graph(G_ba, axes[0])
axes[1].set_title("Degree distribution")
plot_degree_dist(G_ba, axes[1], loglog=False)
if export_figures:
plt.savefig("figures/rand_graph_experiments_2.pdf")
plt.show()
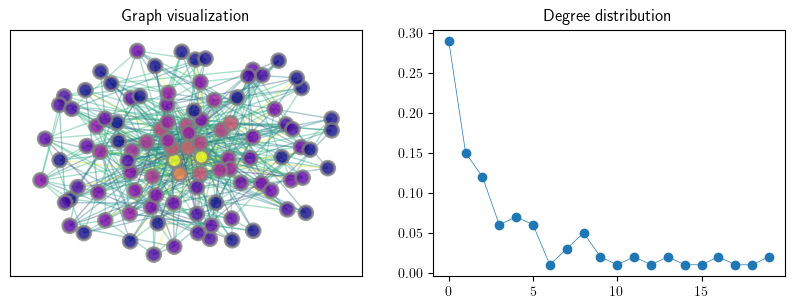